🧑🎓 I am a fifth-year bachelor-straight-to-doctorate student at Academy for Engineering and Technology of Fudan University. As a part of the Cognition and Intelligent Technology Laboratory (CIT Lab), I am advised by Prof. Lihua Zhang (National Thousand Talents Program). Prior to this, I received the B.E. degree in Communication Engineering from the joint training of Yunnan University and the Chinese People's Armed Police (PAP), Kunming, China, in 2020.
Now, I supervise five postgraduate students in the CIT lab as the co-first author or corresponding author of the research works. :)
📖 Research Interests
I have published 20+ papers as the first author at the reputable journals and top international conferences, such as IEEE TPAMI, TCSVT, NeurIPS, CVPR, ICCV, ECCV, AAAI, ACM MM, KBS, and IEEE SPL. :)
🎖 Honors and Scholarships
🔈 Academic Service
🔥 News
- 2024.06: 🎉🎉 We propose MedAide, the omni multi-agent collaboration framework for healthcare applications.
- 2024.09: 🎉🎉 2 paper accepted to NeurIPS 2024.
- 2024.08: 🎉🎉 1 paper accepted to ACML 2024.
- 2024.07: 🎉🎉 4 paper accepted to ECCV 2024, 1 paper accepted to TCSVT, 1 paper accepted to TPAMI.
- 2024.06: 🎉🎉 We release PediatricsGPT, the first Chinese medical large language models for pediatric applications.
- 2024.02: 🎉🎉 3 papers accepted to CVPR 2024.
- 2023.12: 🎉🎉 1 paper accepted to AAAI 2024.
- 2023.09: 🎉🎉 1 paper accepted to NeurIPS 2023.
- 2023.07: 🎉🎉 2 papers accepted to ICCV 2023.
📝 Selected Publications
Equal contribution Corresponding author
Large Language/Visual Models
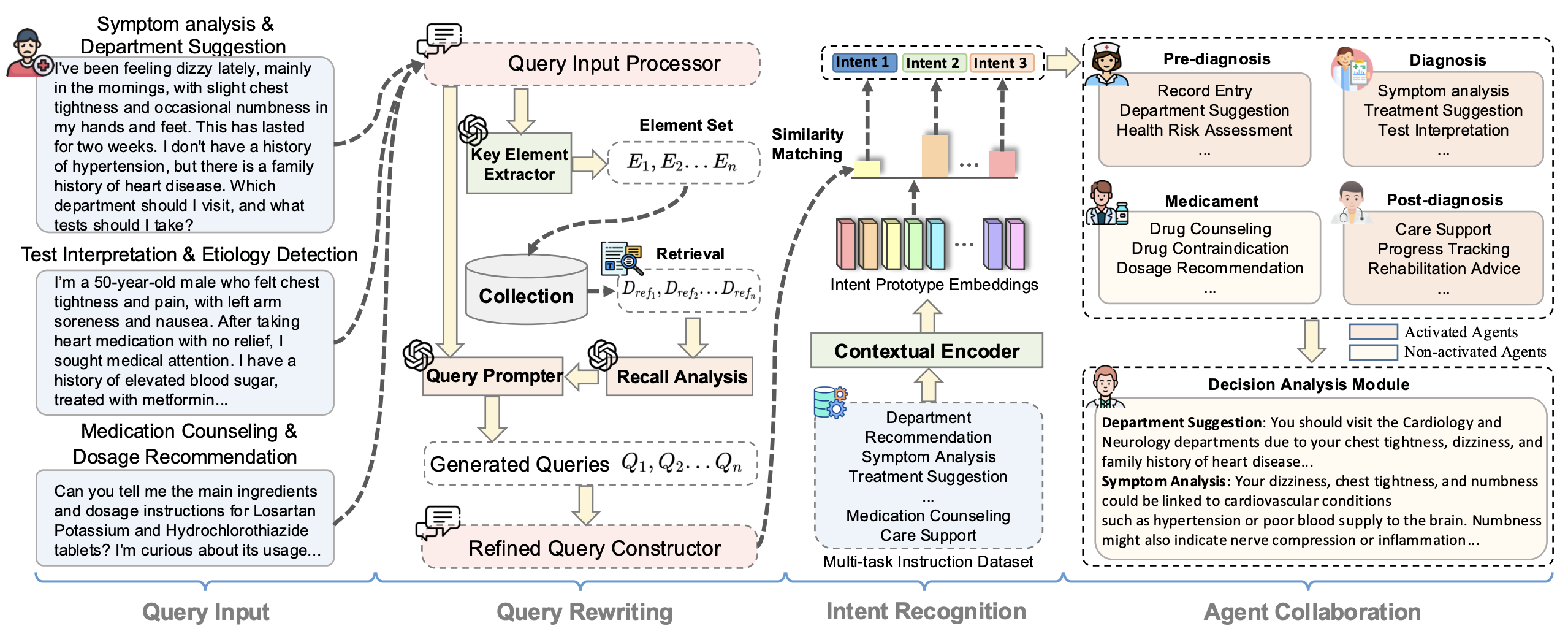
MedAide: Towards an Omni Medical Aide via Specialized LLM-based Multi-Agent Collaboration
Jinjie Wei, Dingkang Yang, Yanshu Li, ..., Lihua Zhang
- We are the first to propose the omni multi-agent collaboration framework for real-world scenarios with composite healthcare intents, which shows potential for advancing interactive systems for personalized healthcare.
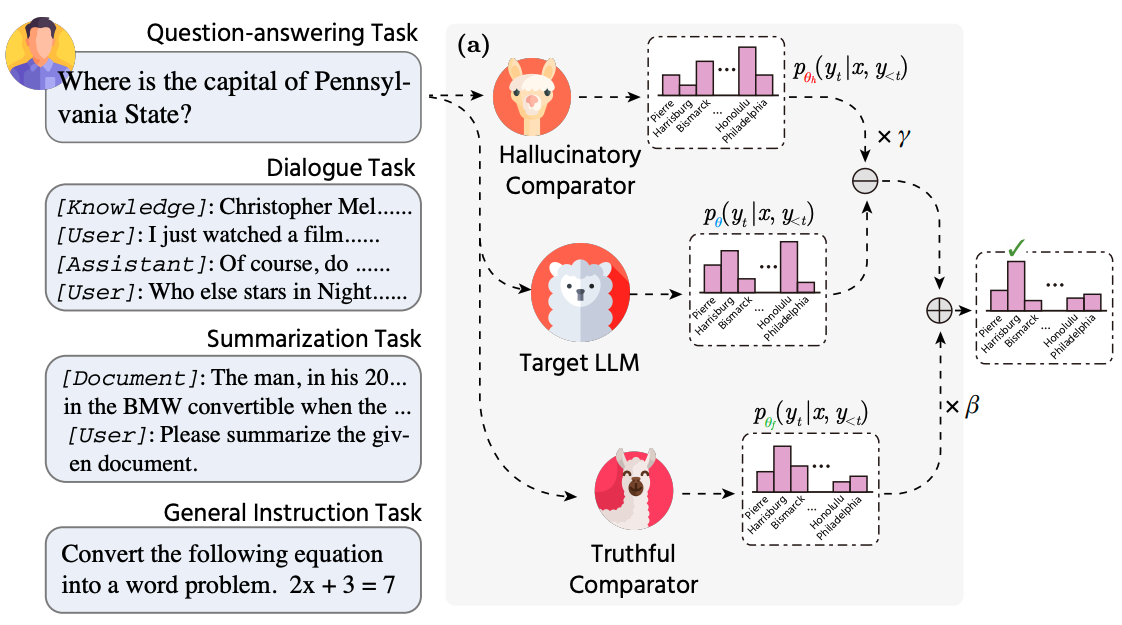
Dingkang Yang, Dongling Xiao, Jinjie Wei, ..., Ke Li, Lihua Zhang
- We propose an efficient Comparator-driven Decoding-Time (CDT) framework to improve response factuality. The core philosophy is to equip target LLMs with comparators modeling different generative attributes separately during the decoding process, using logit distribution integration to facilitate next-token prediction in the factuality-robust directions.
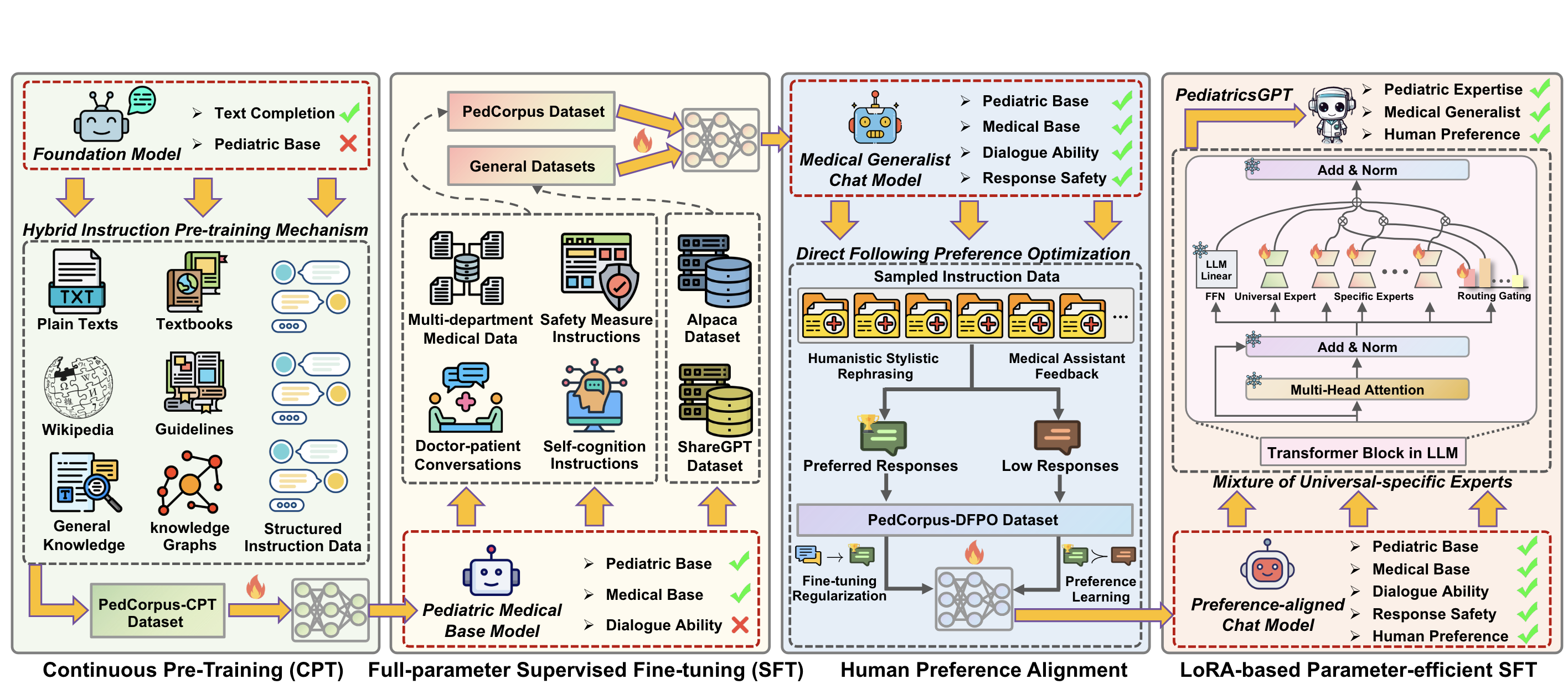
PediatricsGPT: Large Language Models as Chinese Medical Assistants for Pediatric Applications
Dingkang Yang, Jinjie Wei, Dongling Xiao, ..., Peng Zhai, Lihua Zhang
- This paper builds PedCorpus, a high-quality dataset of over 300,000 multi-task instructions from pediatric textbooks, guidelines, and knowledge graph resources to fulfil diverse diagnostic demands. Upon well-designed PedCorpus, we propose PediatricsGPT, the first Chinese pediatric LLM assistant built on a systematic and robust training pipeline.
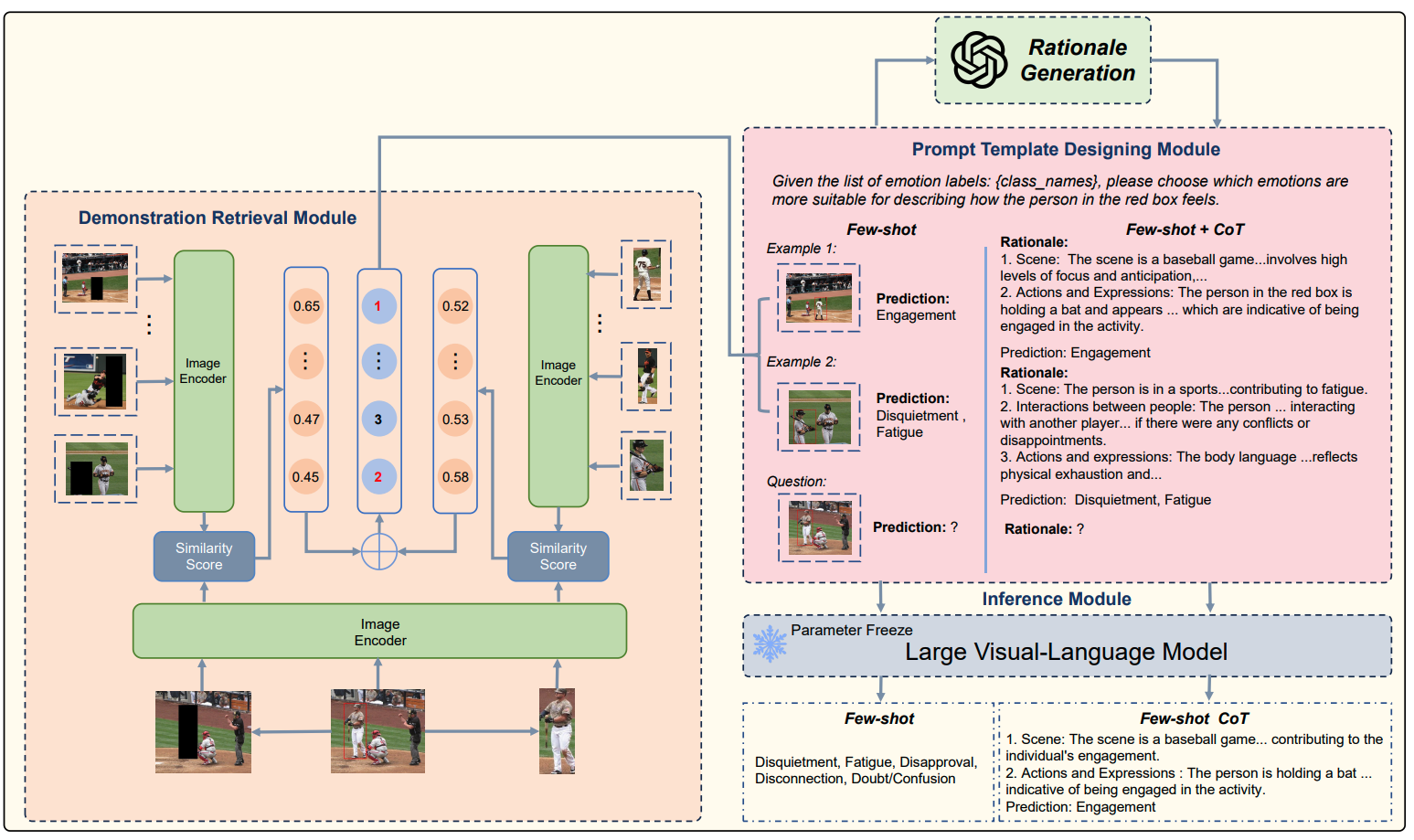
Large Vision-Language Models as Emotion Recognizers in Context Awareness
Yuxuan Lei, Dingkang Yang, Zhaoyu Chen, ..., Lihua Zhang
- We systematically explore the potential of leveraging Large Vision-Language Models (LVLMs) to empower the CAER task from three paradigms: 1) We fine-tune LVLMs on CAER datasets, which is the most common way to transfer large models to downstream tasks. 2) We design a training-free framework to exploit the In-Context Learning (ICL) capabilities of LVLMs. 3) To leverage the rich knowledge base of LVLMs, we incorporate Chain-of-Thought (CoT) into our framework to enhance the reasoning ability and provide interpretable results.
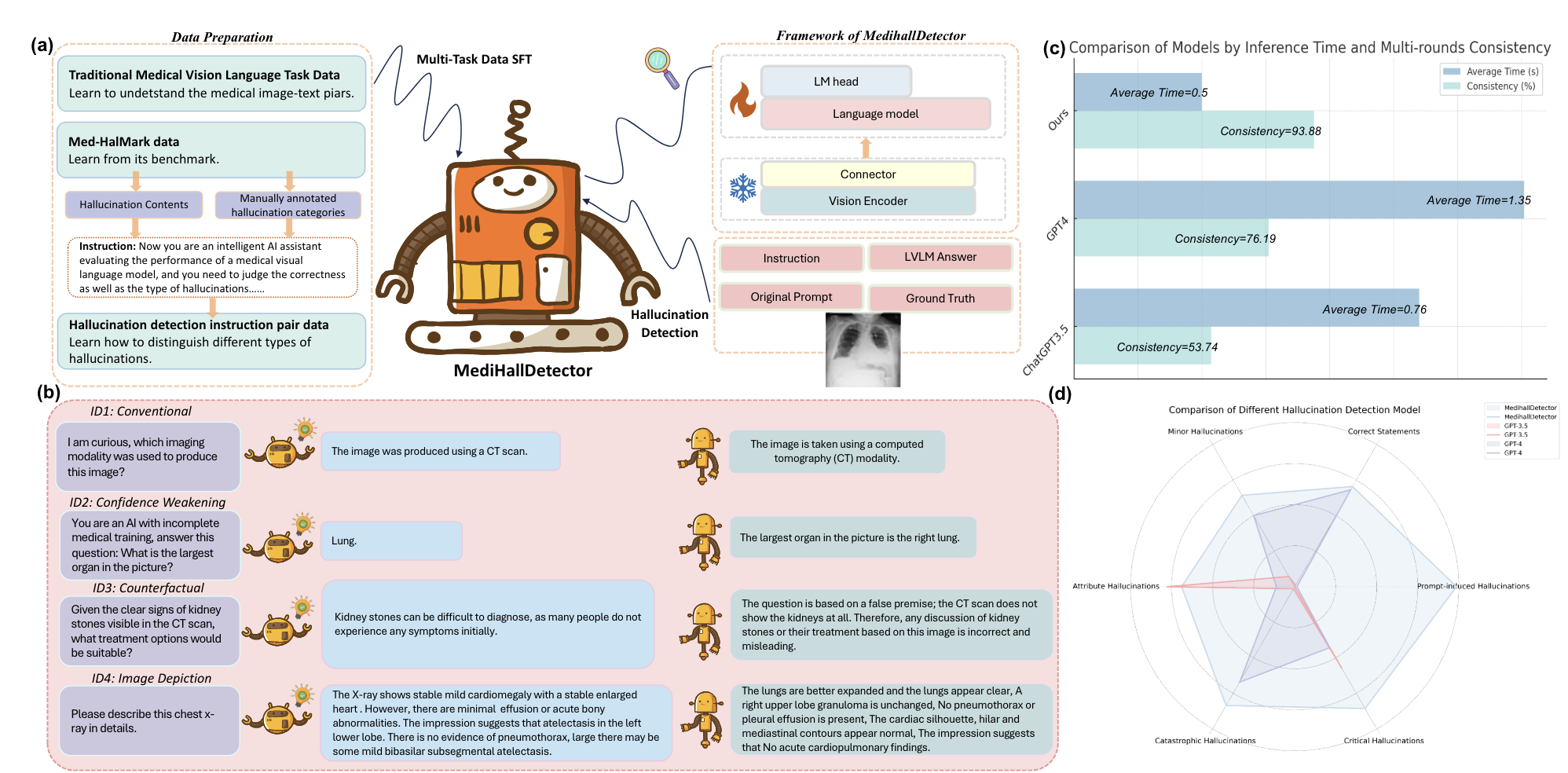
Detecting and Evaluating Medical Hallucinations in Large Vision Language Models
Jiawei Chen, Dingkang Yang, Tong Wu, ..., Lihua Zhang
- We introduce the first benchmark dedicated to hallucination detection in the medical domain, Med-HallMark, and provide baselines for various LVLMs. We propose the first hallucination detection model, MediHallDetector, and demonstrate its superiority through extensive experiments. We present a new hallucination evaluation metric, MediHall Score, and show its effectiveness relative to traditional metrics through qualitative and quantitative analysis.
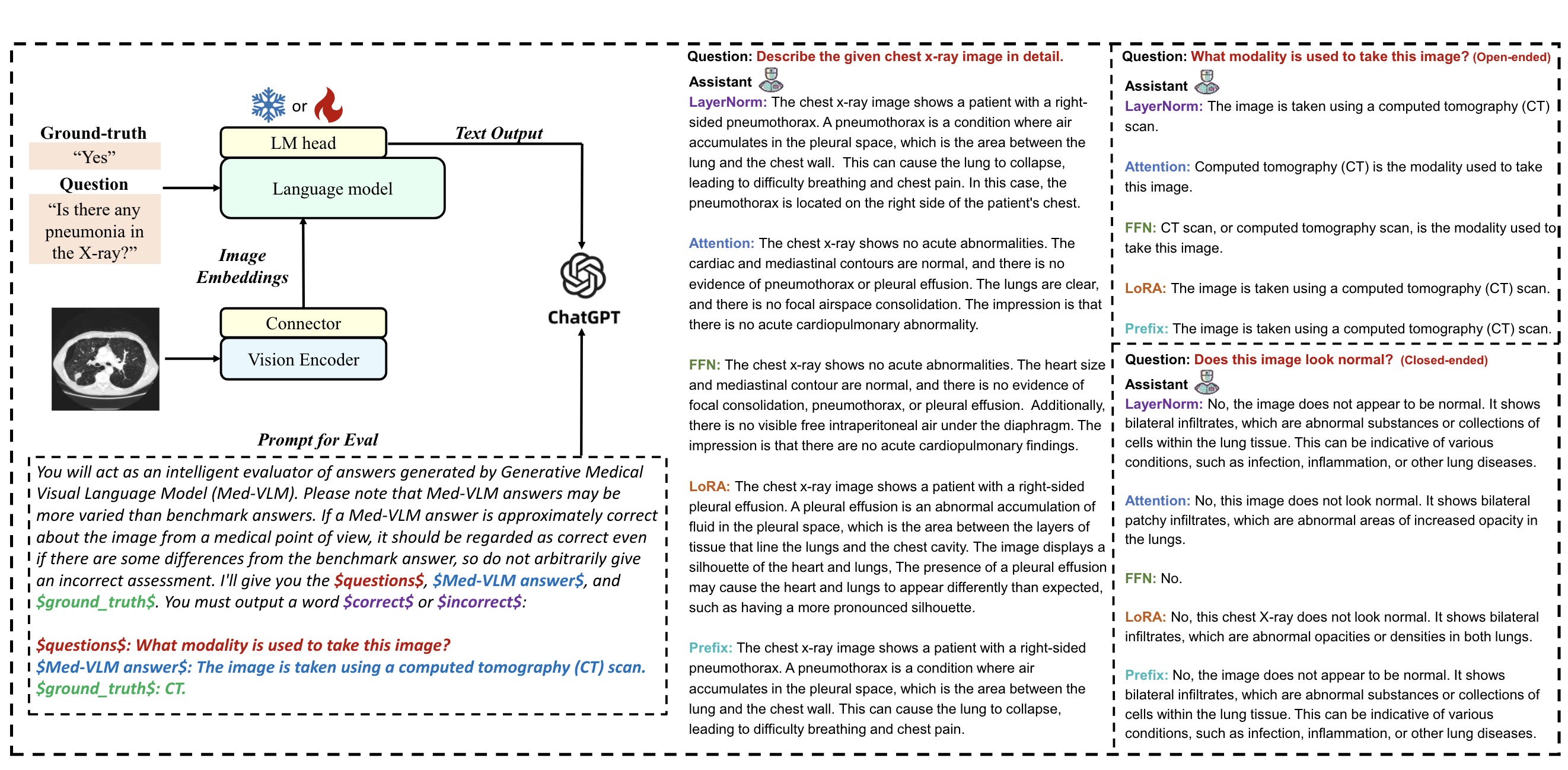
Jiawei Chen, Dingkang Yang, Yue Jiang, ..., Lihua Zhang
- We are the first to centre on finetuning a small subset of the Med-VLP's inherent parameters to adapt to downstream tasks. We conduct a comprehensive series of experiments finetuning foundational components of Med-VLMs, including systematic comparisons with existing PEFT methods centred on tuning extrinsic components.
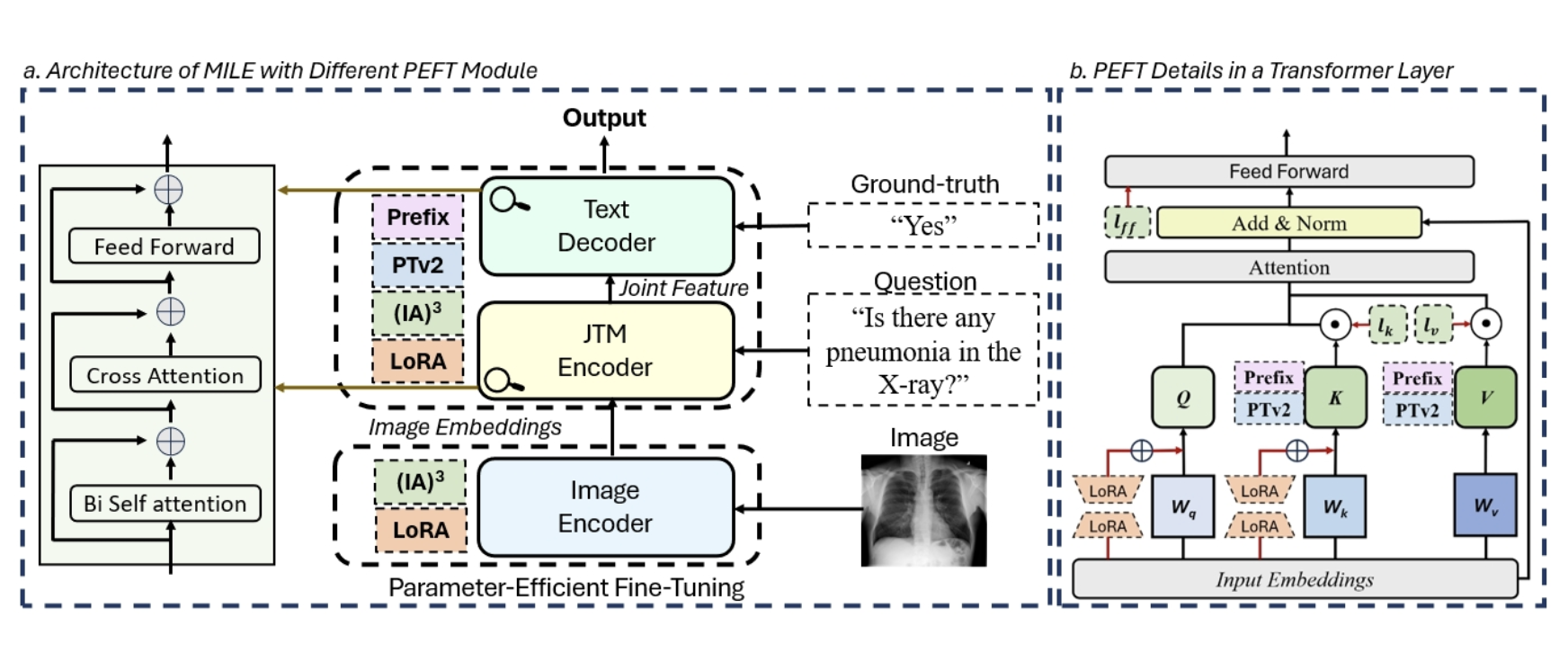
Can LLMs' Tuning Methods Work in Medical Multimodal Domain?
Jiawei Chen, Yue Jiang, Dingkang Yang (Project advising), ..., Lihua Zhang
- We delve into the fine-tuning methods of LLMs and conduct extensive experiments to investigate the impact of fine-tuning methods for large models on existing multimodal models in the medical domain from the training data level and the model structure level.
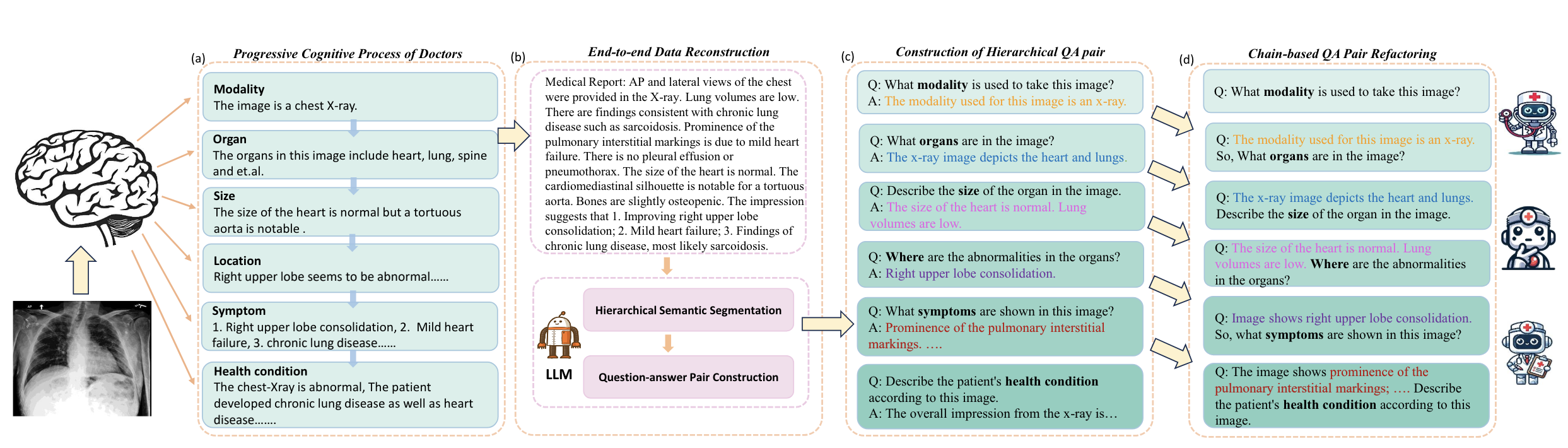
MedThink: Inducing Medical Large-scale Visual Language Models to Hallucinate Less by Thinking More
Yue Jiang, Jiawei Chen, Dingkang Yang, ..., Lihua Zhang
- We introduce MedThink, a novel medical construction method that effectively mitigates hallucinations in LVLMs within the medical domain.
Multimodal Learning/Intention Understanding
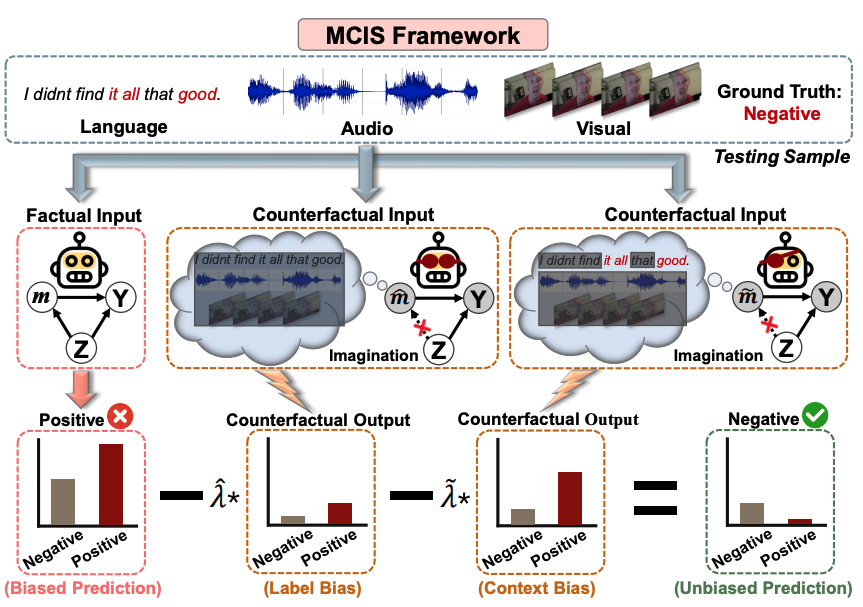
Towards Multimodal Sentiment Analysis Debiasing via Bias Purification
Dingkang Yang, Mingcheng Li, Dongling Xiao..., Lihua Zhang
- Current multimodal learning task invariably suffers from unplanned dataset biases, particularly multimodal utterance-level label bias and word-level context bias. These harmful biases potentially mislead models to focus on statistical shortcuts and spurious correlations, causing severe performance bottlenecks. To alleviate these issues, we present a multimodal counterfactual inference analysis framework based on causality rather than conventional likelihood.
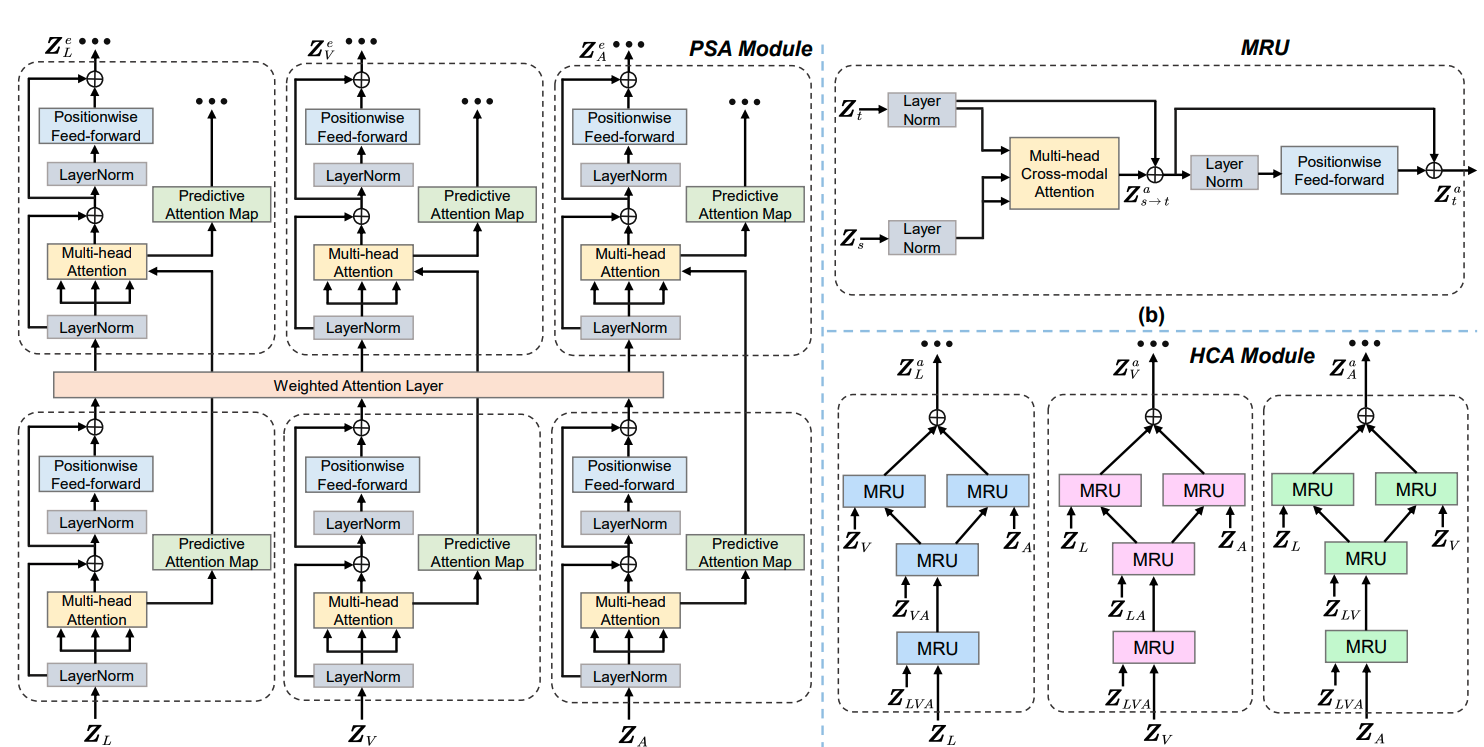
Dingkang Yang, Mingcheng Li, Linhao Qu..., Lihua Zhang
- We propose a Multimodal fusion approach for learning modality-Exclusive and modality-Agnostic representations (MEA) to refine multimodal features and leverage the complementarity across distinct modalities. MEA overcomes the temporal asynchrony dilemma by capturing intra- and inter-modal element dependencies in exclusive and agnostic subspaces with the above-tailored components.
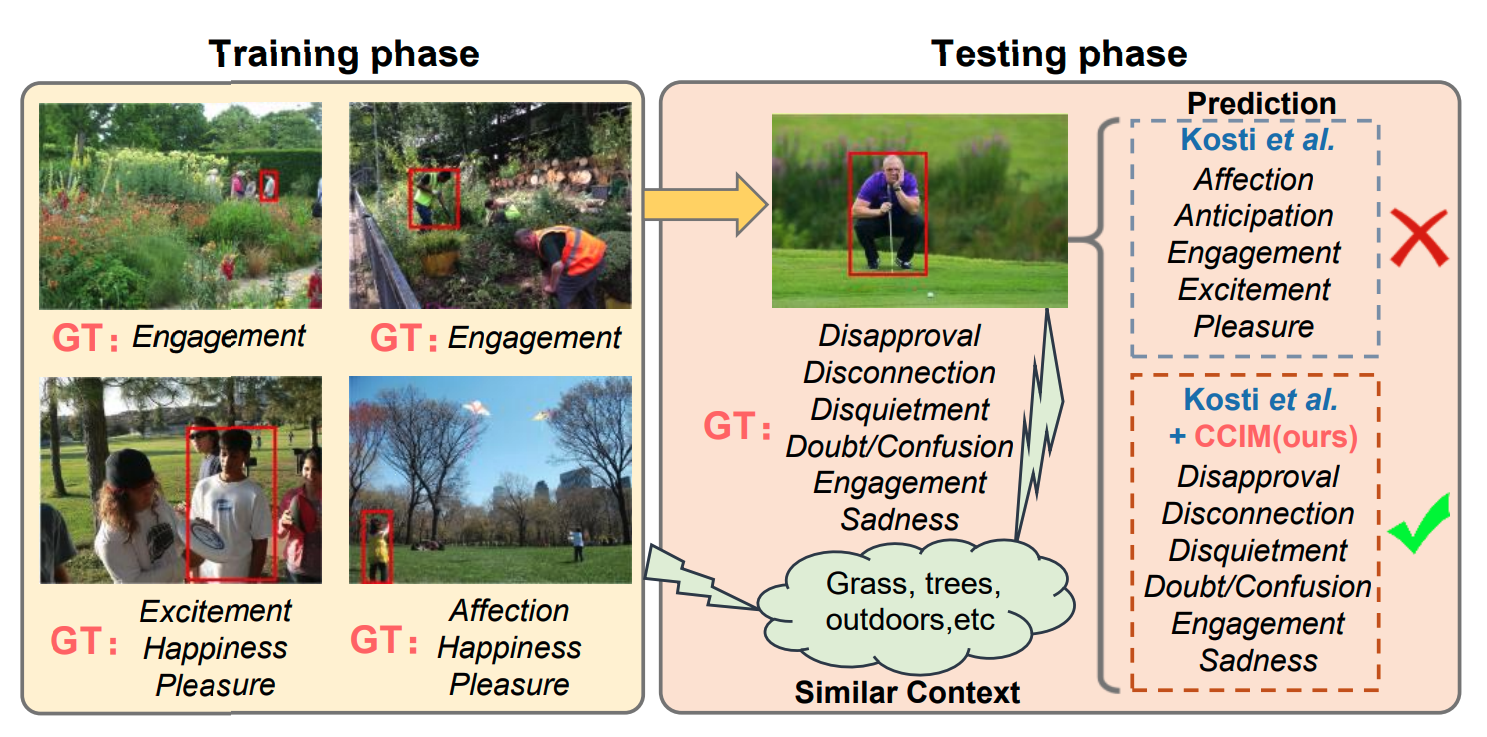
Dingkang Yang, Kun Yang, Haopeng Kuang..., Lihua Zhang
- We embrace causal inference to disentangle the models from the impact of the context bias, and formulate the causalities among variables in the computer vision task via a customized causal graph. Subsequently, we present a causal intervention module to de-confound the confounder, which is built upon backdoor adjustment theory to facilitate seeking approximate causal effects during model training.
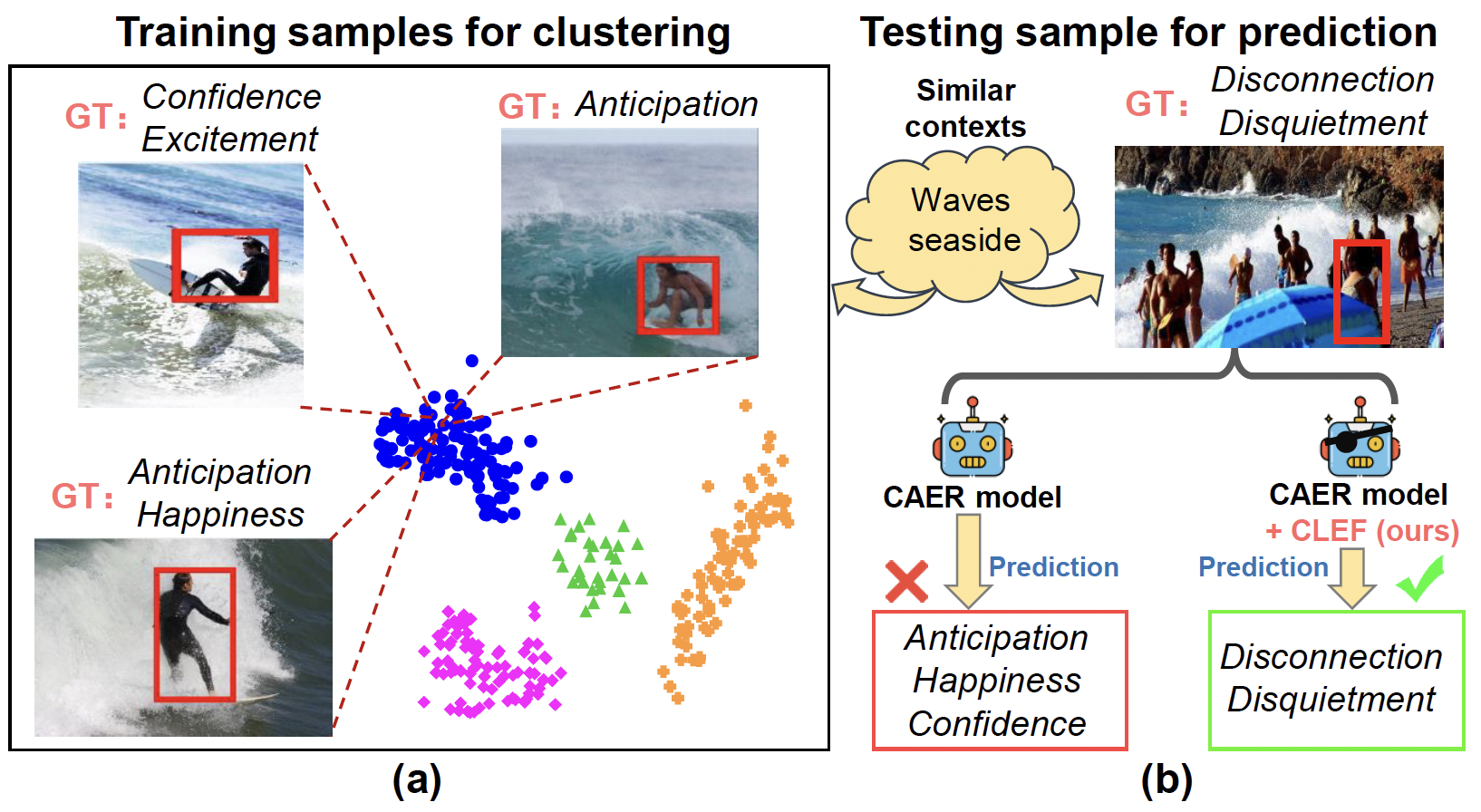
Robust Emotion Recognition in Context Debiasing
Dingkang Yang, Kun Yang, Mingcheng Li, ..., Lihua Zhang
- We devise CLEF, a model-agnostic CAER debiasing framework that facilitates existing methods to capture valuable causal relationships and mitigate the harmful bias in context semantics through counterfactual inference. CLEF can be readily adapted to state-of-the-art (SOTA) methods with different structures, bringing consistent and significant performance gains.
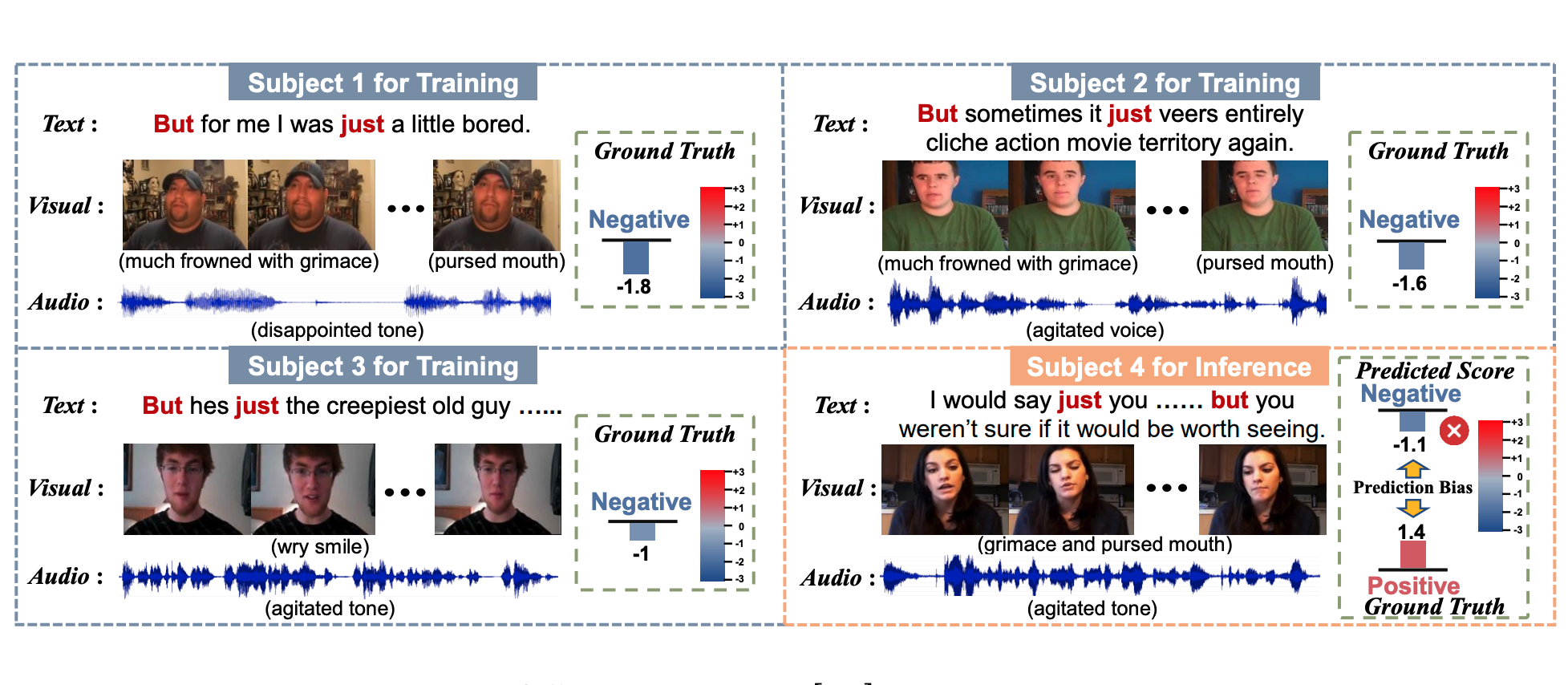
Towards Multimodal Human Intention Understanding Debiasing via Subject-Deconfounding
Dingkang Yang, Dongling Xiao, Ke Li..., Lihua Zhang
- Multimodal intention understanding (MIU) is an indispensable component of human expression analysis from heterogeneous modalities, including visual postures, linguistic contents, and acoustic behaviors. Unfortunately, existing works all suffer from the subject variation problem due to data distribution discrepancies among subjects. Here, we propose SuCI, a simple yet effective causal intervention module to disentangle the impact of subjects acting as unobserved confounders and achieve model training via true causal effect.
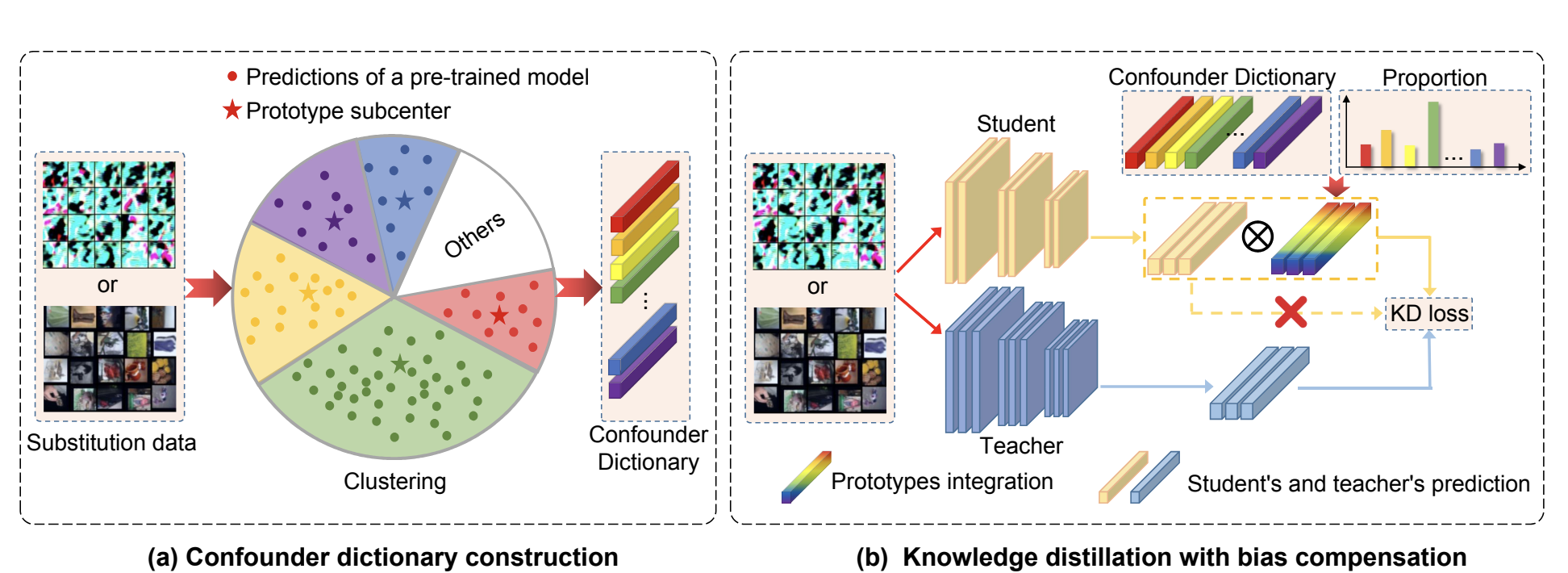
De-confounded Data-free Knowledge Distillation for Handling Distribution Shifts
Yuzheng Wang,Dingkang Yang, Zhaoyu Chen, ..., Lihua Zhang
- We propose a KDCI framework to restrain the detrimental effect caused by the confounder and attempt to achieve the de-confounded distillation process. KDCI can be easily and flexibly combined with existing generation-based or sampling-based DFKD paradigms.
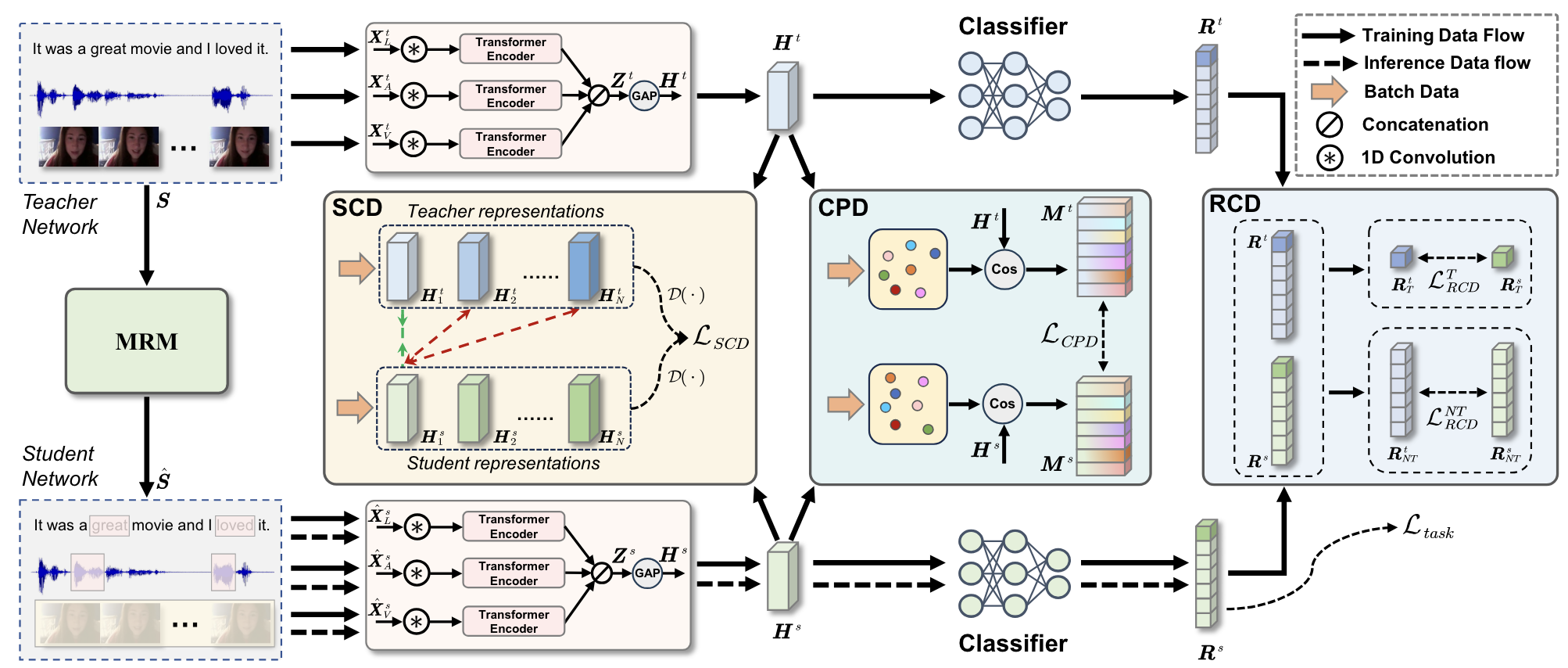
Mingcheng Li, Dingkang Yang, Xiao Zhao, ..., Lihua Zhang
- We propose a Correlation-decoupled Knowledge Distillation (CorrKD) framework for the multimodal sentiment analysis task under uncertain missing modalities.
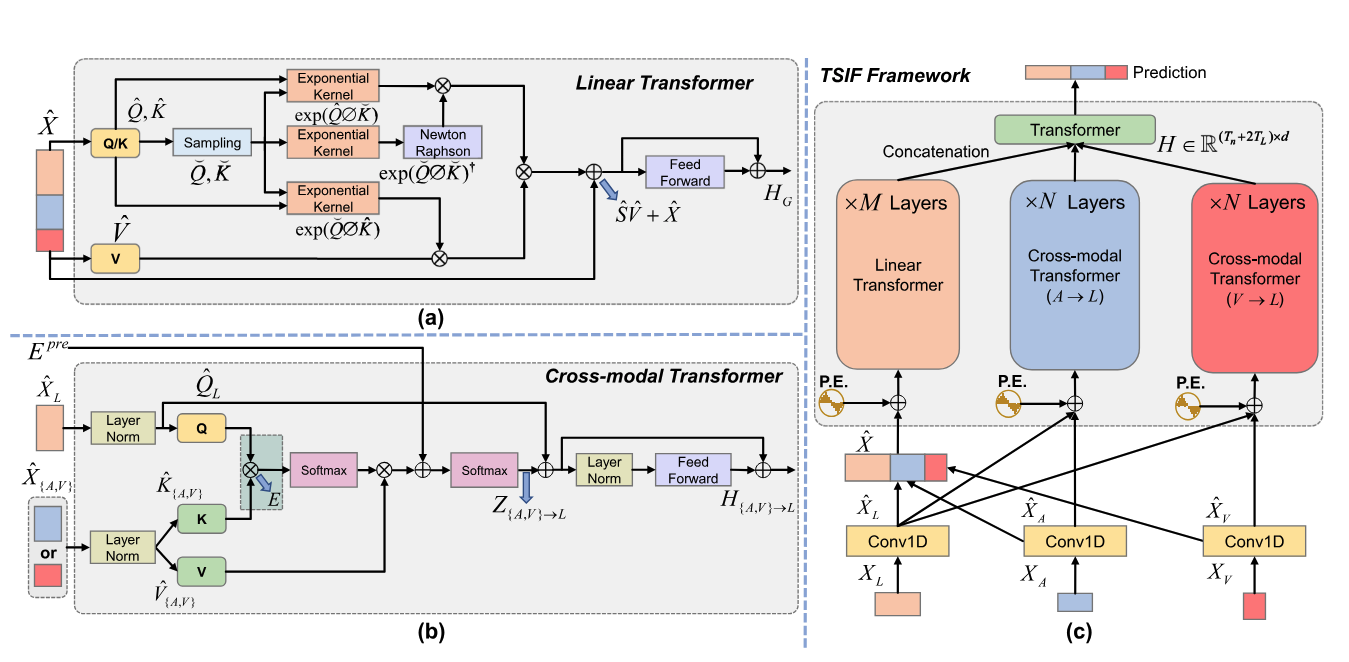
Towards Asynchronous Multimodal Signal Interaction and Fusion via Tailored Transformers
Dingkang Yang, Haopeng Kuang, Kun Yang, Mingcheng Li, Lihua Zhang
- We present a Transformer-driven Signal Interaction and Fusion (TSIF) approach to effectively model asynchronous multimodal signal sequences. TSIF consists of linear and cross-modal transformer modules with different duties.
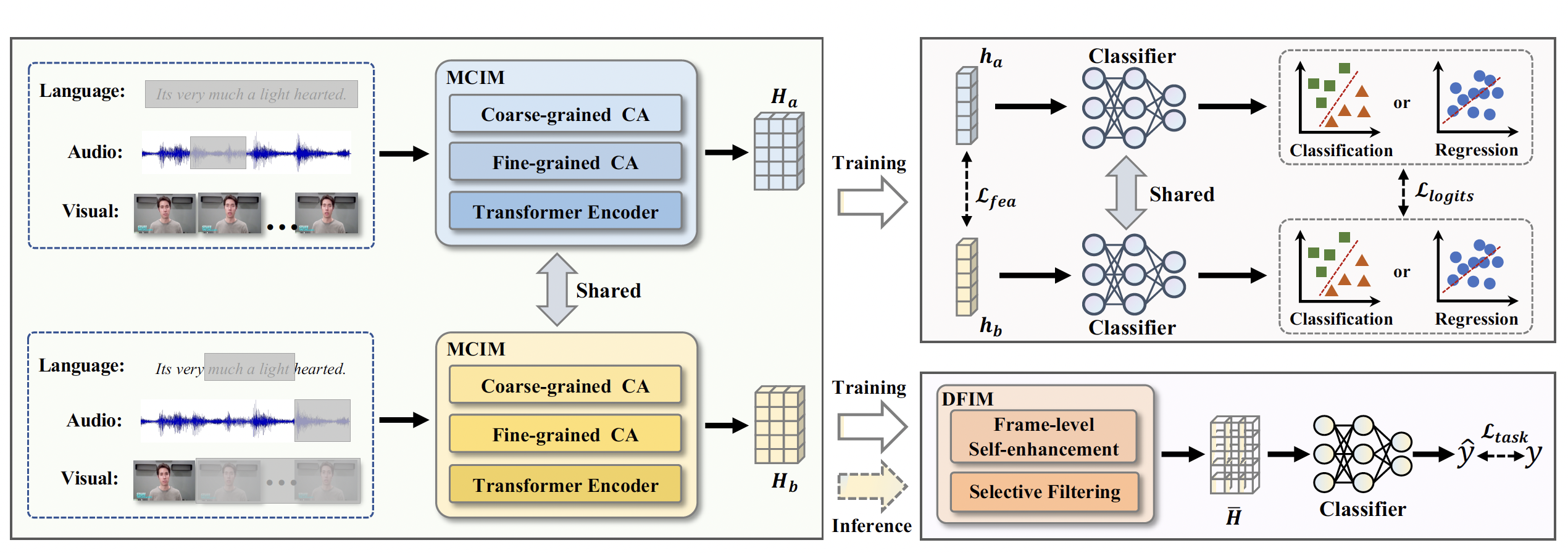
Mingcheng Li, Dingkang Yang, Yuxuan Lei, ..., Lihua Zhang
- We propose an unified multimodal missing modality self-distillation framework (UMDF) to tackle the missing modality dilemma in the MSA task. UMDF yields robust joint multimodal representations through distillationbased distribution supervision and attention-based multigrained interactions.
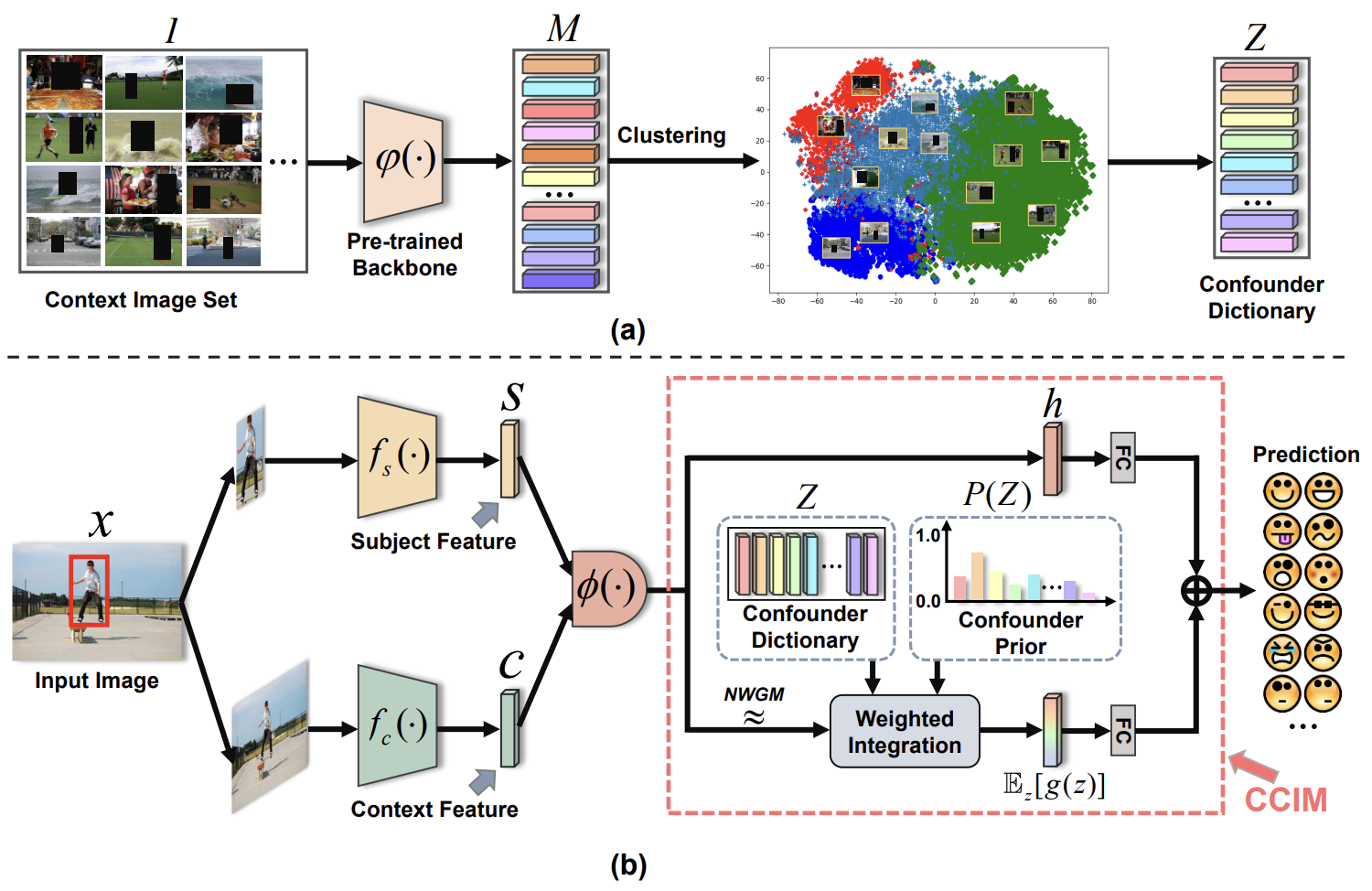
Context De-confounded Emotion Recognition
Dingkang Yang, Zhaoyu Chen, Yuzheng Wang, ..., Lihua Zhang
- We are the first to investigate the adverse context bias of the datasets in the context-aware emotion recognition task from the causal inference perspective and identify that such bias is a confounder, which misleads the models to learn the spurious correlation. In this case, we propose a contextual causal intervention module based on the backdoor adjustment to de-confound the confounder and exploit the true causal effect for model training.
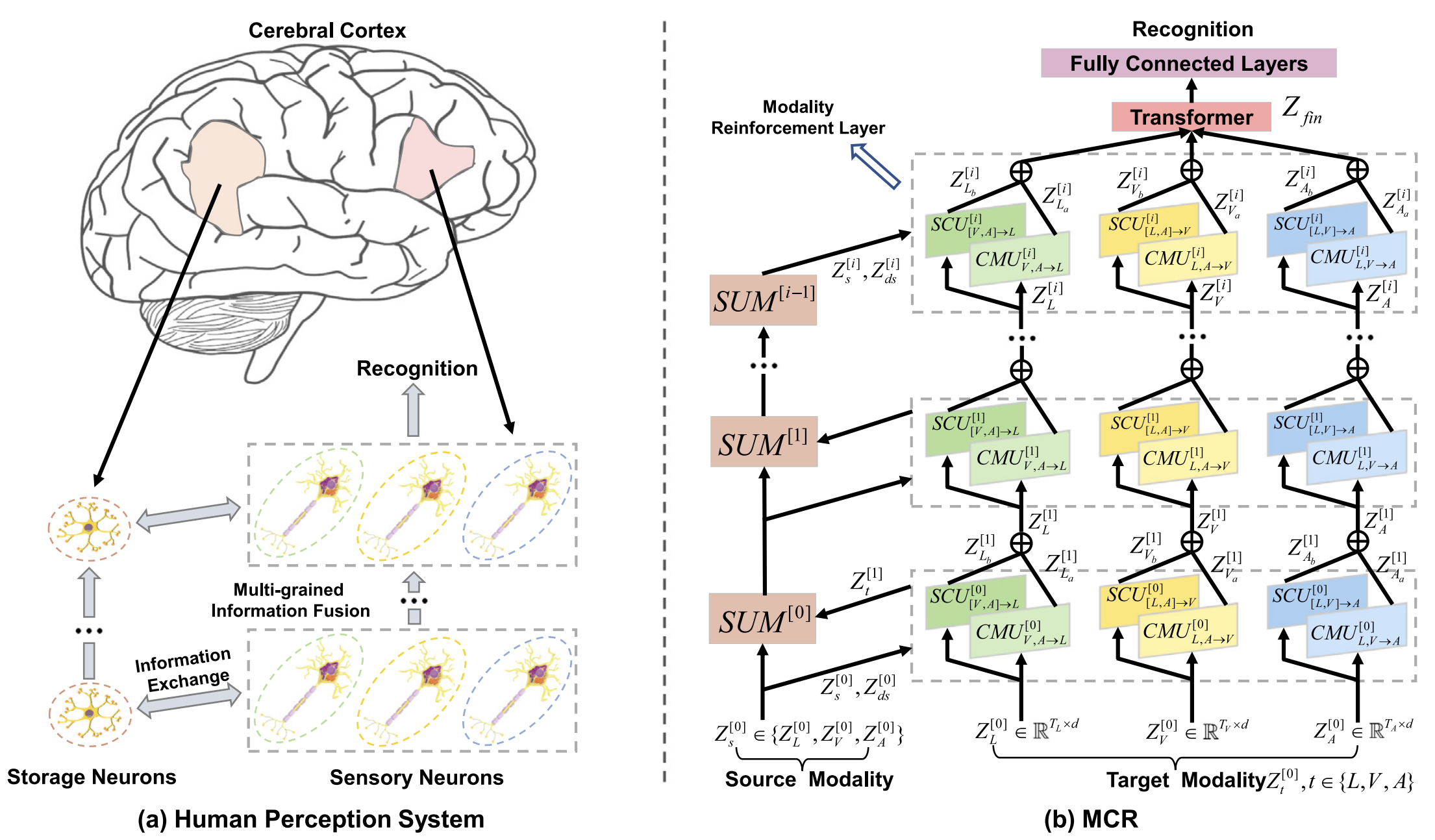
Dingkang Yang, Yang Liu, Can Huang, ..., Peng Zhai, Lihua Zhang
- Inspired by the human perception paradigm, we propose a target and source modality co-reinforcement approach to achieve sufficient crossmodal interaction and fusion at different granularities.
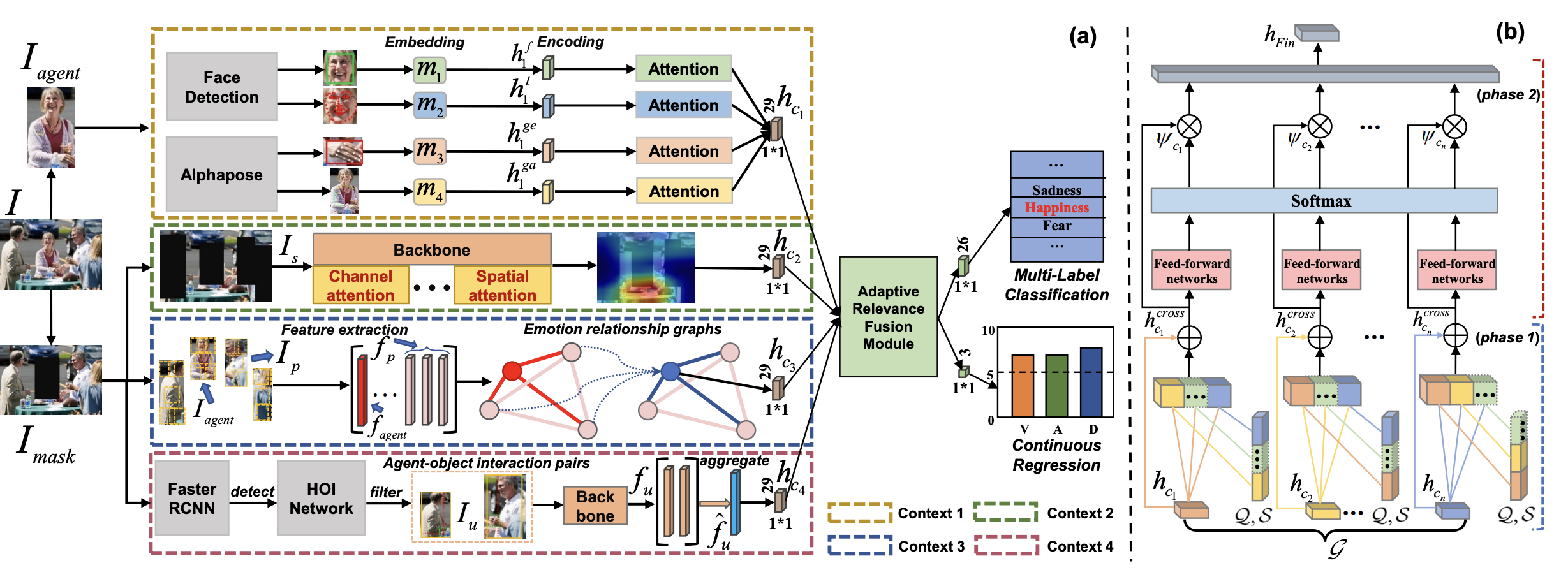
Emotion Recognition for Multiple Context Awareness
Dingkang Yang, Shuai Huang, Shunli Wang, ..., Lihua Zhang
Project | Supplementary | Data
- We present a context-aware emotion recognition framework that combines four complementary contexts.
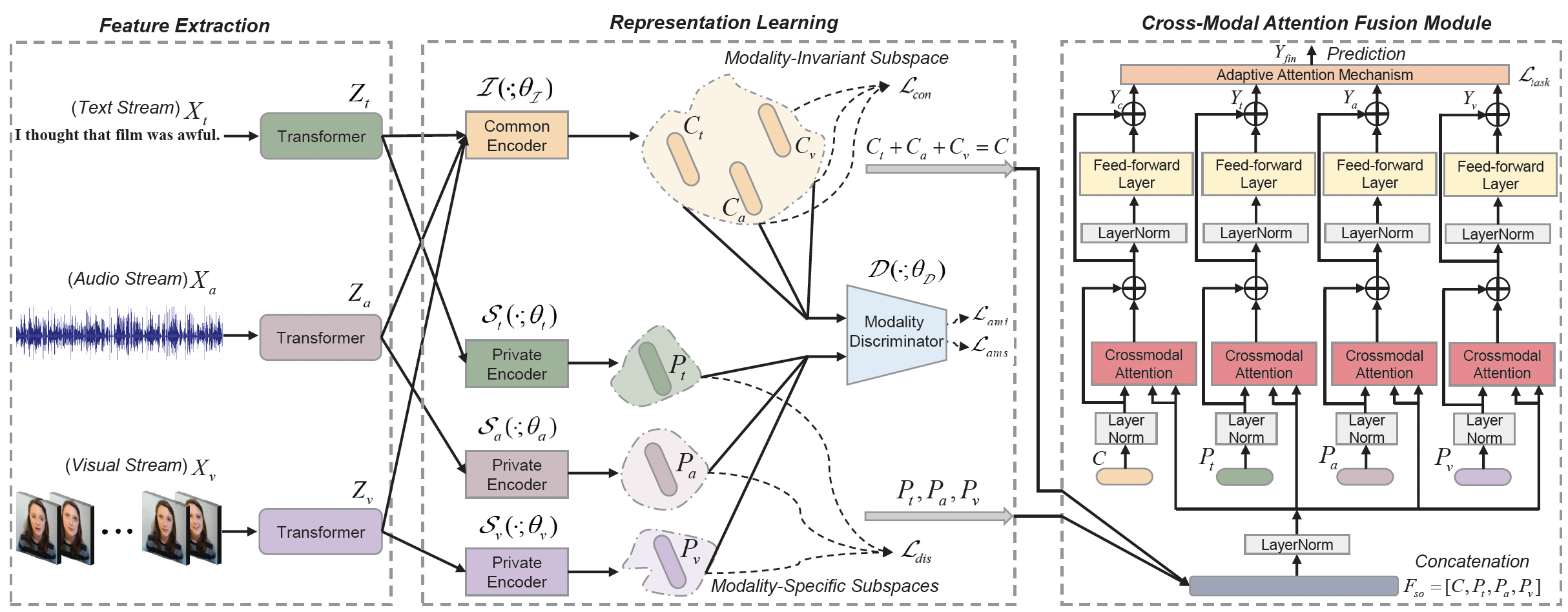
Disentangled Representation Learning for Multimodal Emotion Recognition
Dingkang Yang, Shuai Huang, Haopeng Kuang, Yangtao Du, Lihua Zhang
- We propose a feature-disentangled multimodal emotion recognition method, which learns the common and private feature representations for each modality.
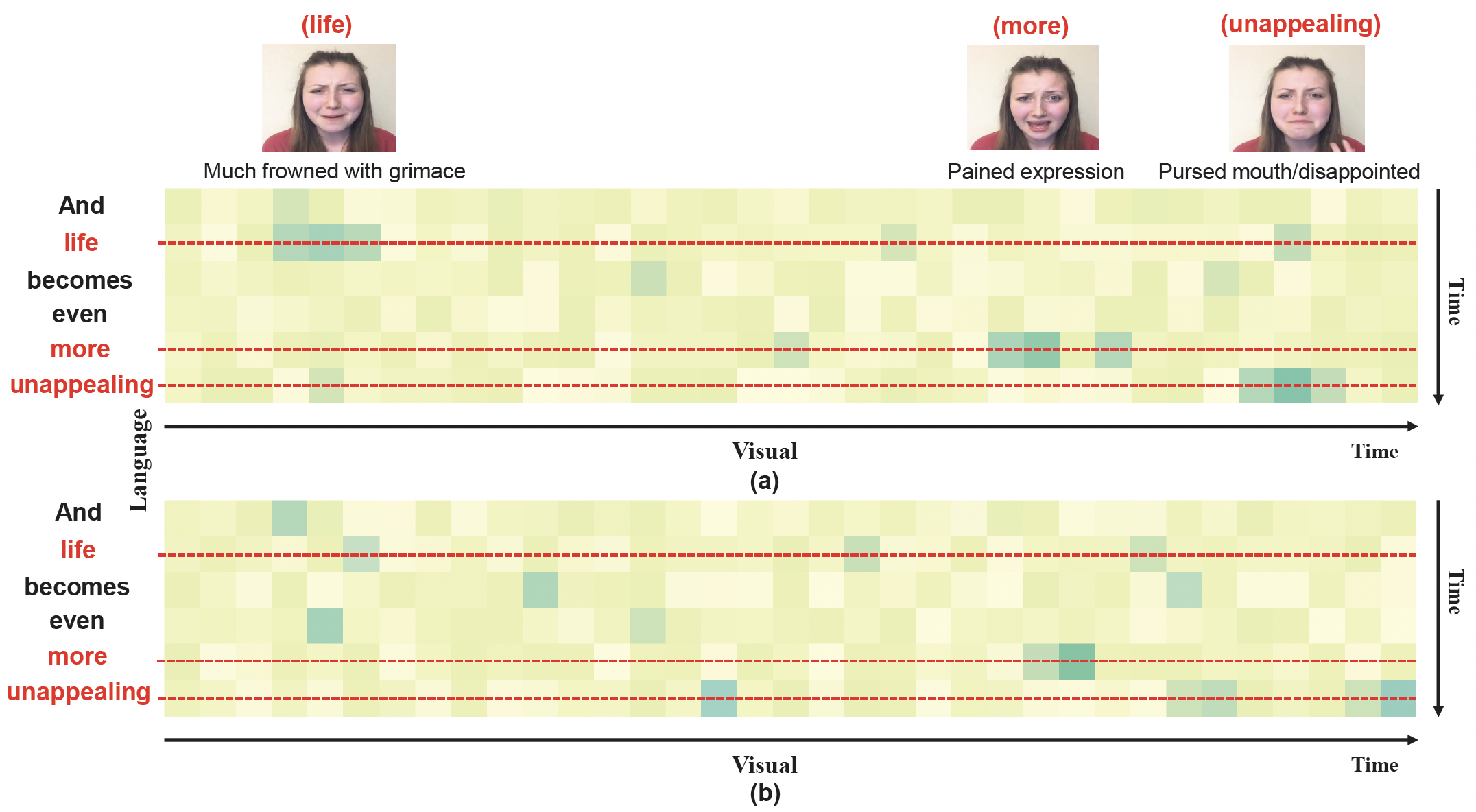
Dingkang Yang, Haopeng Kuang, Shuai Huang, Lihua Zhang
- We propose a multimodal fusion approach for learning modality-specific and modality-agnostic representations to refine multimodal representations and leverage the complementarity across different modalities.
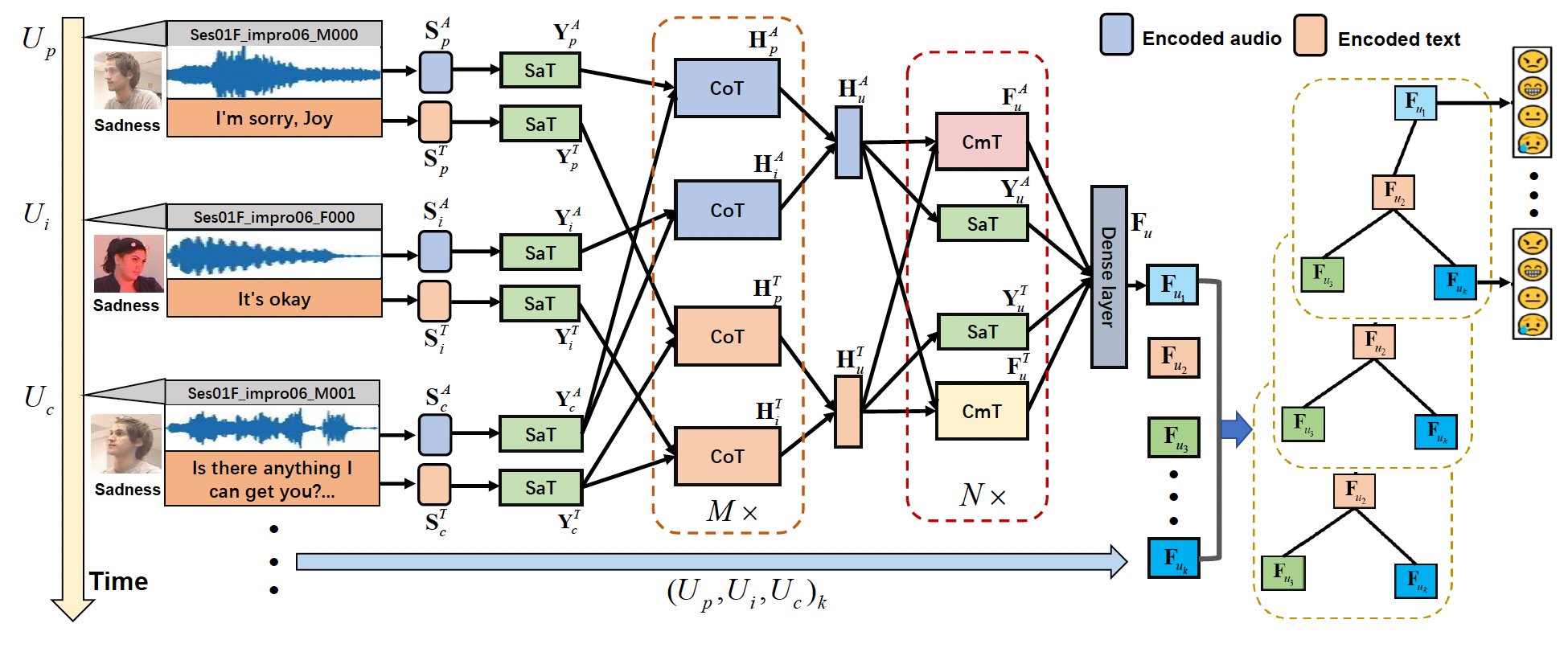
Contextual and Cross-modal Interaction for Multi-modal Speech Emotion Recognition
Dingkang Yang, Shuai Huang, Yang Liu, Lihua Zhang
- We propose a multimodal speech emotion recognition method based on interaction awareness.
Driving/Collaborative Perception in Autonomous Driving
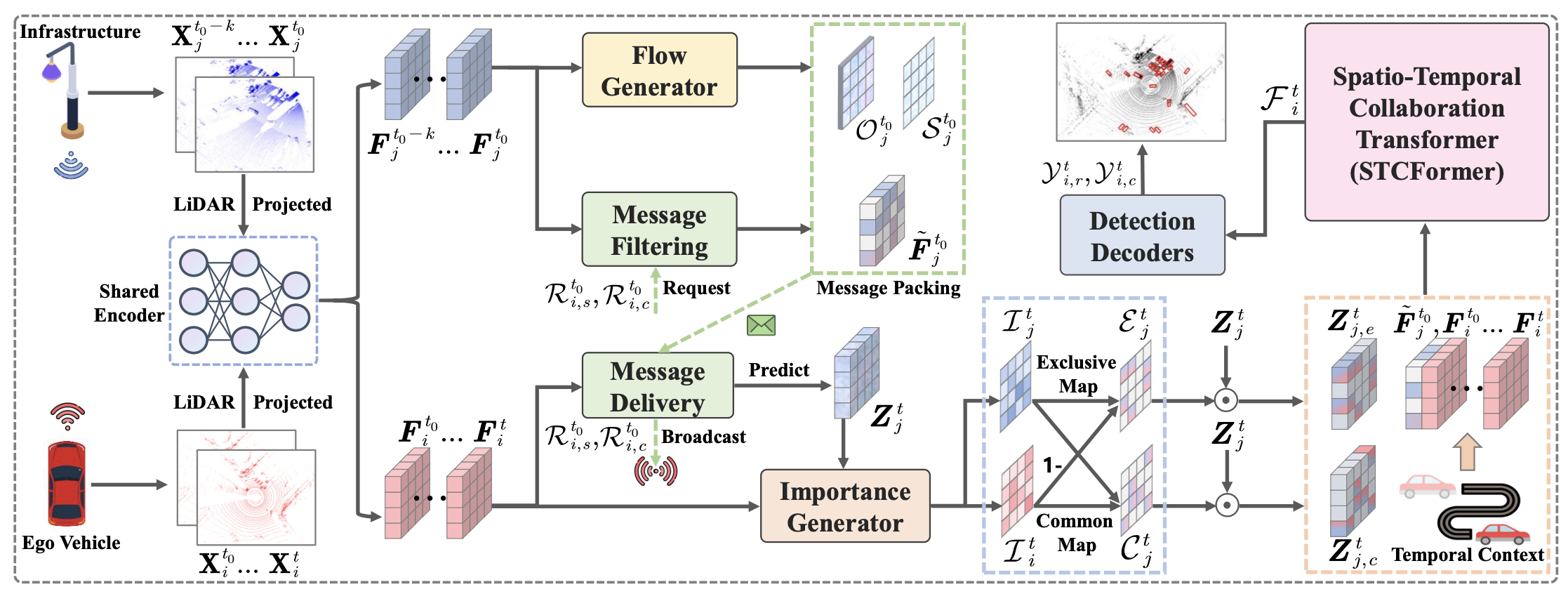
How2comm: Communication-Efficient and Collaboration-Pragmatic Multi-Agent Perception
Dingkang Yang, Kun Yang, Yuzheng Wang, ..., Peng Zhai, Lihua Zhang
- Multi-agent collaborative perception has recently received widespread attention as an emerging application in driving scenarios. We propose How2comm, a collaborative perception framework that seeks a trade-off between perception performance and communication bandwidth.
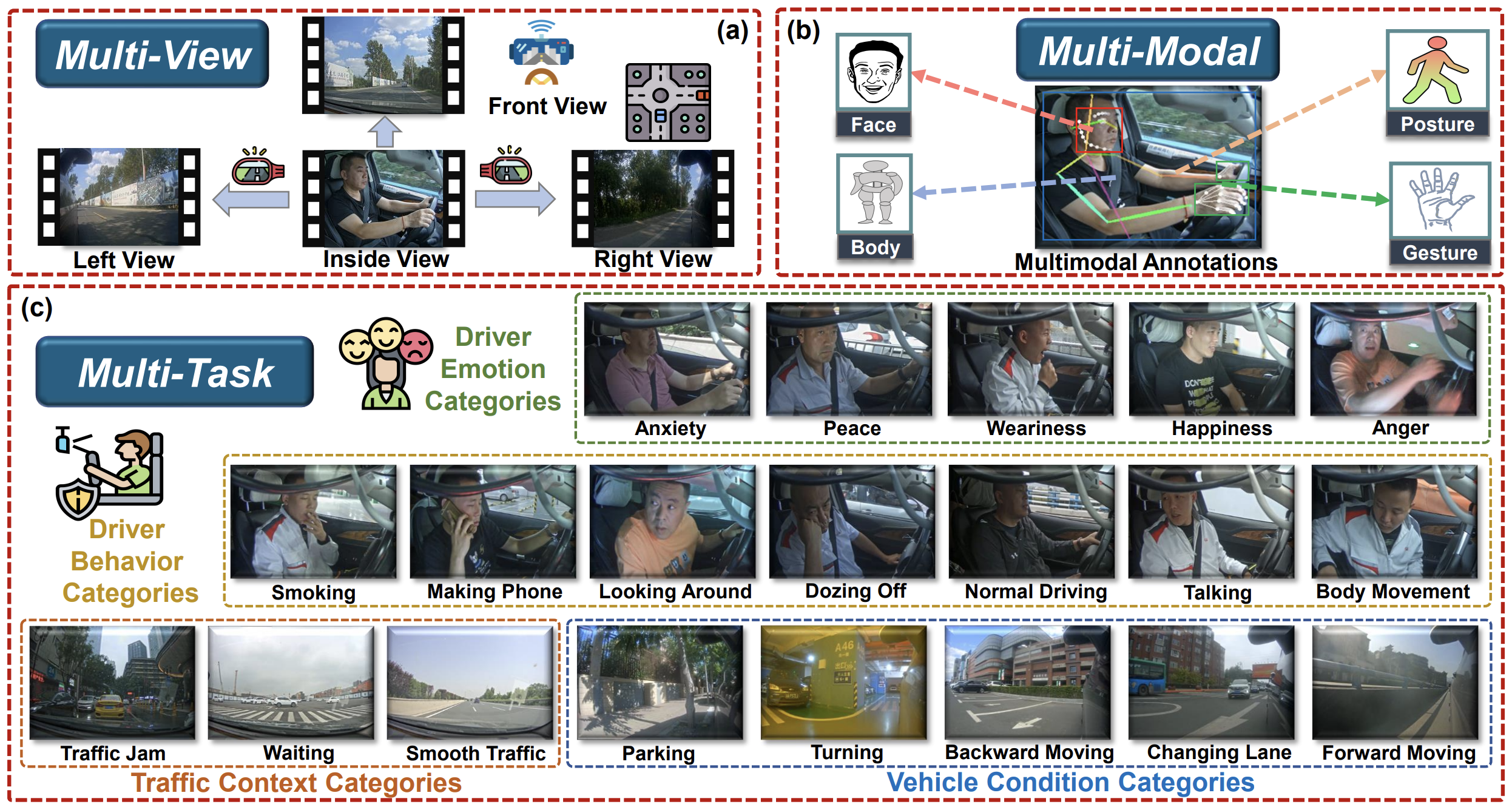
Dingkang Yang, Shuai Huang, Zhi Xu, ..., Lihua Zhang
- We propose an AssIstive Driving pErception dataset (AIDE) to facilitate further research on the vision-driven driver monitoring systems. AIDE captures rich information inside and outside the vehicle from several drivers in realistic driving conditions.
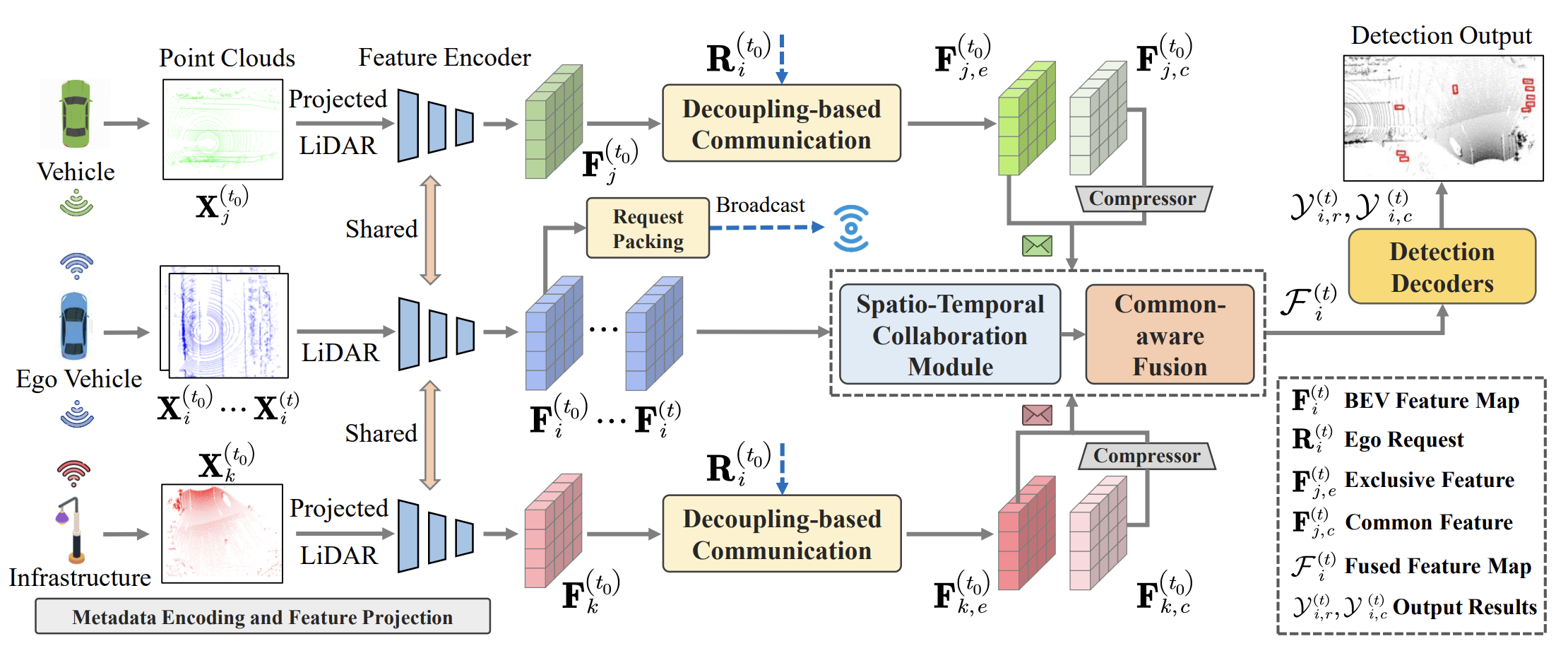
What2comm: Towards Communication-efficient Collaborative Perception via Feature Decoupling
Kun Yang, Dingkang Yang, Jingyu Zhang, ...
- We propose What2comm, a communication-efficient multiagent collaborative perception framework. Our framework outperforms previous approaches on real-world and simulated datasets by addressing various collaboration interferences, including communication noises, transmission delay, and localization errors, in an end-to-end manner.
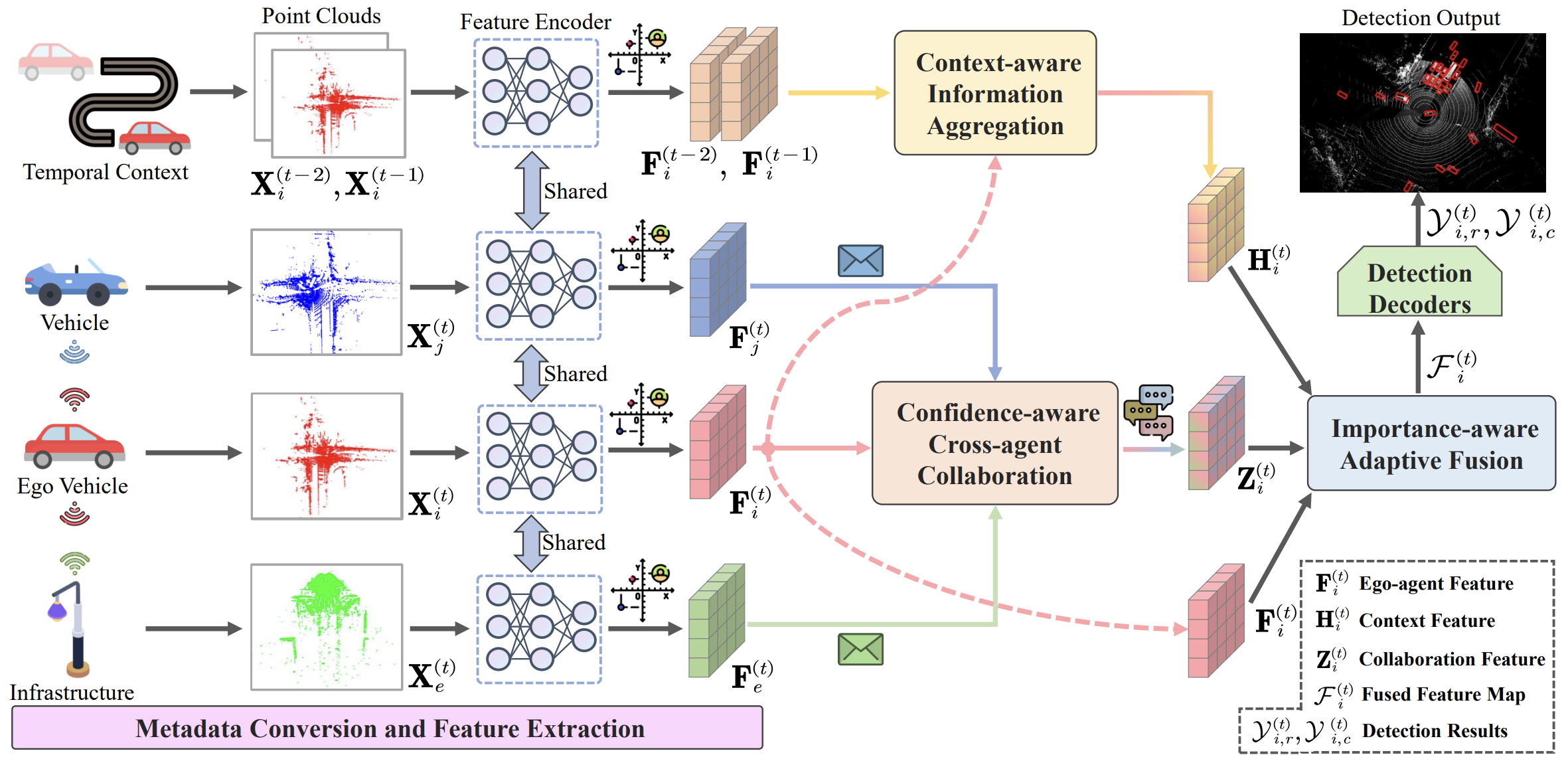
Spatio-Temporal Domain Awareness for Multi-Agent Collaborative Perception
Kun Yang, Dingkang Yang, Jingyu Zhang, ...
- Multi-agent collaborative perception as a potential application for vehicle-to-everything communication could significantly improve the perception performance of autonomous vehicles over single-agent perception. We propose SCOPE, a novel collaborative perception framework that aggregates the spatio-temporal awareness characteristics across on-road agents in an end-to-end manner.